Policy or Value ? Loss Function and Playing Strength in AlphaZero
Por um escritor misterioso
Last updated 14 abril 2025
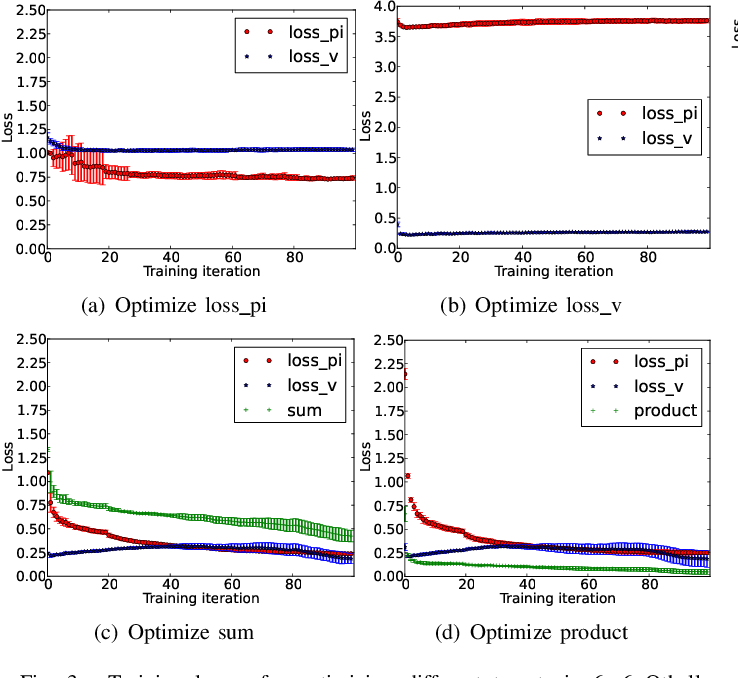
Results indicate that, at least for relatively simple games such as 6x6 Othello and Connect Four, optimizing the sum, as AlphaZero does, performs consistently worse than other objectives, in particular by optimizing only the value loss. Recently, AlphaZero has achieved outstanding performance in playing Go, Chess, and Shogi. Players in AlphaZero consist of a combination of Monte Carlo Tree Search and a Deep Q-network, that is trained using self-play. The unified Deep Q-network has a policy-head and a value-head. In AlphaZero, during training, the optimization minimizes the sum of the policy loss and the value loss. However, it is not clear if and under which circumstances other formulations of the objective function are better. Therefore, in this paper, we perform experiments with combinations of these two optimization targets. Self-play is a computationally intensive method. By using small games, we are able to perform multiple test cases. We use a light-weight open source reimplementation of AlphaZero on two different games. We investigate optimizing the two targets independently, and also try different combinations (sum and product). Our results indicate that, at least for relatively simple games such as 6x6 Othello and Connect Four, optimizing the sum, as AlphaZero does, performs consistently worse than other objectives, in particular by optimizing only the value loss. Moreover, we find that care must be taken in computing the playing strength. Tournament Elo ratings differ from training Elo ratings—training Elo ratings, though cheap to compute and frequently reported, can be misleading and may lead to bias. It is currently not clear how these results transfer to more complex games and if there is a phase transition between our setting and the AlphaZero application to Go where the sum is seemingly the better choice.
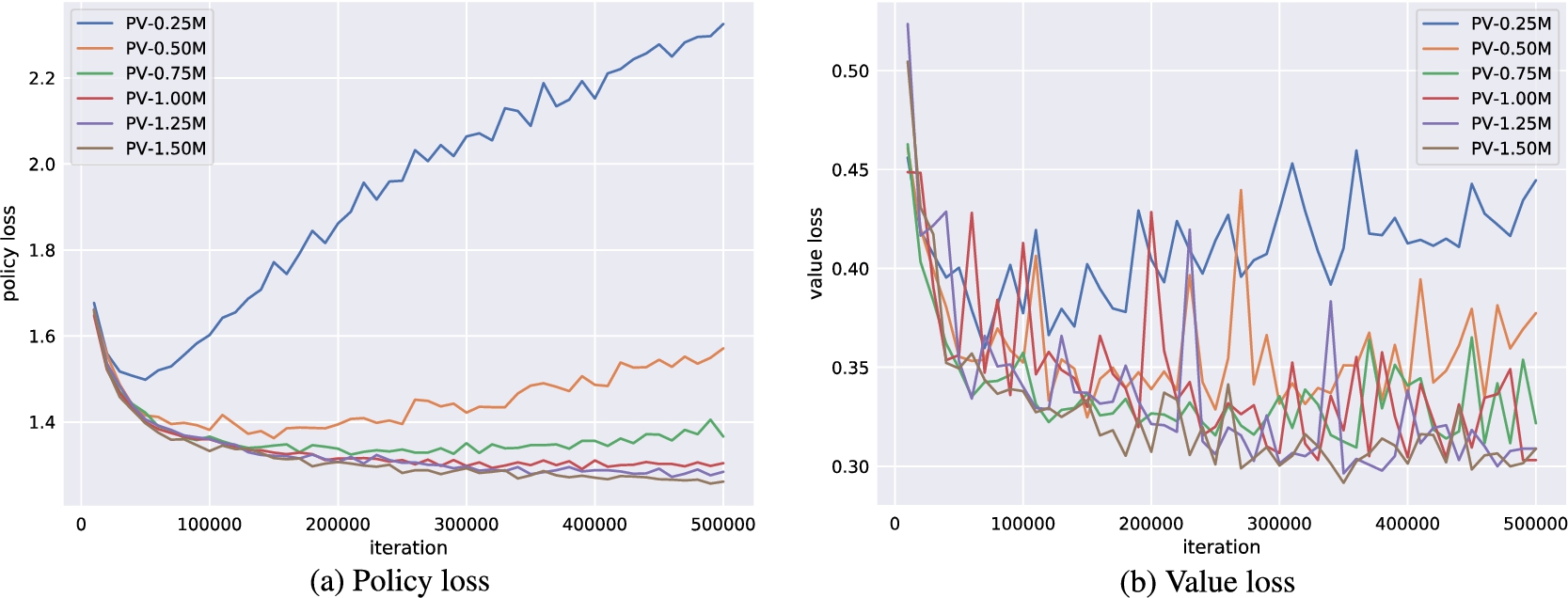
RankNet for evaluation functions of the game of Go - IOS Press

AlphaGo Zero – How and Why it Works – Tim Wheeler
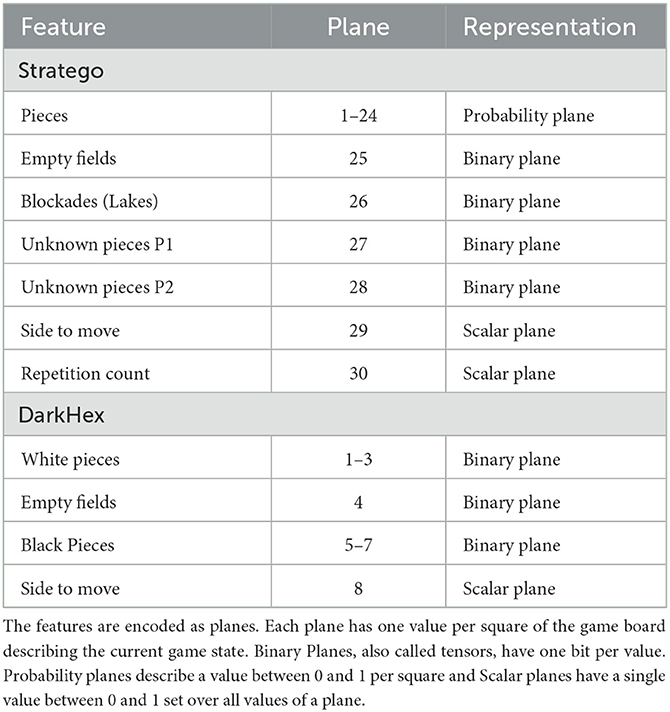
Frontiers AlphaZe∗∗: AlphaZero-like baselines for imperfect information games are surprisingly strong

AlphaGo Zero – How and Why it Works – Tim Wheeler

AlphaGo Zero – How and Why it Works – Tim Wheeler

AlphaZero, Vladimir Kramnik and reinventing chess

Reimagining Chess with AlphaZero, February 2022
The future is here – AlphaZero learns chess
Simple Alpha Zero

AlphaZero

Student of Games: A unified learning algorithm for both perfect and imperfect information games
Recomendado para você
-
Checkmate: how we mastered the AlphaZero cover, Science14 abril 2025
-
Revista de Xadrez New In Chess 2019-8 Magnus Carlsen Observe as Fotos14 abril 2025
-
How DeepMind's AlphaGo Became the World's Top Go Player, by Andre Ye14 abril 2025
-
AlphaZero: DeepMind's New Chess AI14 abril 2025
-
STREET FIGHTER ALPHA ZERO RYU ANIME PRODUCTION CEL 614 abril 2025
-
Alpha S 2 Pickleball Paddle Bundle - Pickleball Paddle Shop14 abril 2025
-
Free Course: DeepMind's AlphaGo Zero and AlphaZero, RL paper explained from Aleksa Gordić - The AI Epiphany14 abril 2025
-
A general reinforcement learning algorithm that masters chess, shogi, and Go through self-play14 abril 2025
-
AlphaZero paper peer-reviewed is available · Issue #2069 · leela-zero/leela- zero · GitHub14 abril 2025
-
PDF] Reproducibility via Crowdsourced Reverse Engineering: A Neural Network Case Study With DeepMind's Alpha Zero14 abril 2025
você pode gostar
-
Leidse sleutels van de Meelfabriek getakeld14 abril 2025
-
Quadro Decorativo Em Caixa De Acrílico Serena Iii14 abril 2025
-
Bethesda University Christian University in Orange County, CA14 abril 2025
-
Humble Choice Humble Bundle14 abril 2025
-
Conta De Fortnite - Epic Games - DFG14 abril 2025
-
Player who got out Uno reverse card after getting yellow card speaks out14 abril 2025
-
Block Game - collect the blocks - Free download and software reviews - CNET Download14 abril 2025
-
luluca jogando roblox|TikTok Search14 abril 2025
-
Torino x Atalanta: onde assistir, horário, escalações e estatísticas14 abril 2025
-
Minato Namikaze - Desenho de ton_uzumaki_br - Gartic14 abril 2025