Remote Sensing, Free Full-Text
Por um escritor misterioso
Last updated 25 abril 2025
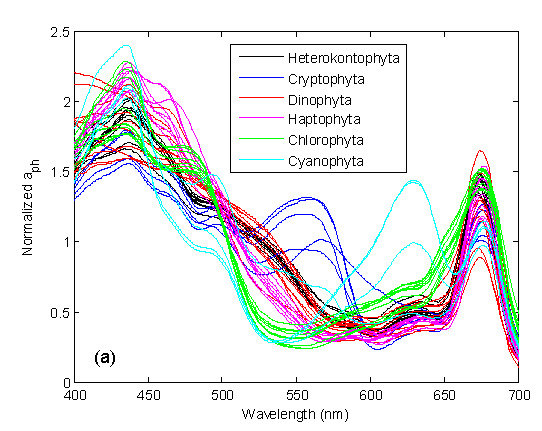
The emergence of hyperspectral optical satellite sensors for ocean observation provides potential for more detailed information from aquatic ecosystems. The German hyperspectral satellite mission EnMAP (enmap.org) currently in the production phase is supported by a project to explore the capability of using EnMAP data and other future hyperspectral data from space. One task is to identify phytoplankton taxonomic groups. To fulfill this objective, on the basis of laboratory-measured absorption coefficients of phytoplankton cultures (aph(λ)) and corresponding simulated remote sensing reflectance spectra (Rrs(λ)), we examined the performance of spectral fourth-derivative analysis and clustering techniques to differentiate six taxonomic groups. We compared different sources of input data, namely aph(λ), Rrs(λ), and the absorption of water compounds obtained from inversion of the Rrs(λ)) spectra using a quasi-analytical algorithm (QAA). Rrs(λ) was tested as it can be directly obtained from hyperspectral sensors. The last one was tested as expected influences of the spectral features of pure water absorption on Rrs(λ) could be avoided after subtracting it from the inverted total absorption. Results showed that derivative analysis of measured aph(λ) spectra performed best with only a few misclassified cultures. Based on Rrs(λ) spectra, the accuracy of this differentiation decreased but the performance was partly restored if wavelengths of strong water absorption were excluded and chlorophyll concentrations were higher than 1 mg∙m−3. When based on QAA-inverted absorption spectra, the differentiation was less precise due to loss of information at longer wavelengths. This analysis showed that, compared to inverted absorption spectra from restricted inversion models, hyperspectral Rrs(λ) is potentially suitable input data for the differentiation of phytoplankton taxonomic groups in prospective EnMAP applications, though still a challenge at low algal concentrations.
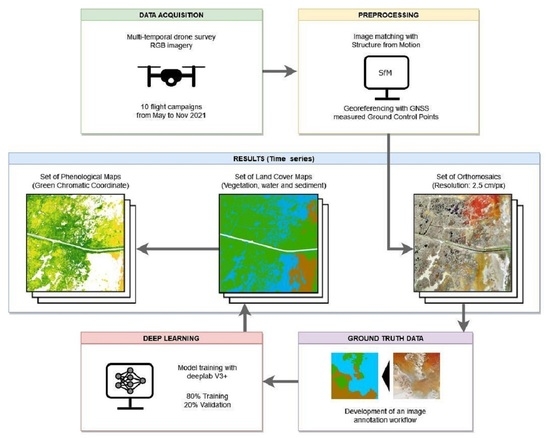
Arcgis 10.1 Tutorial-Daten Herunterladen - Colaboratory

PDF) Integration Review of National Remote Sensing Ground Station Based on Virtual Ground Station by Full Remote and Nearly Automation
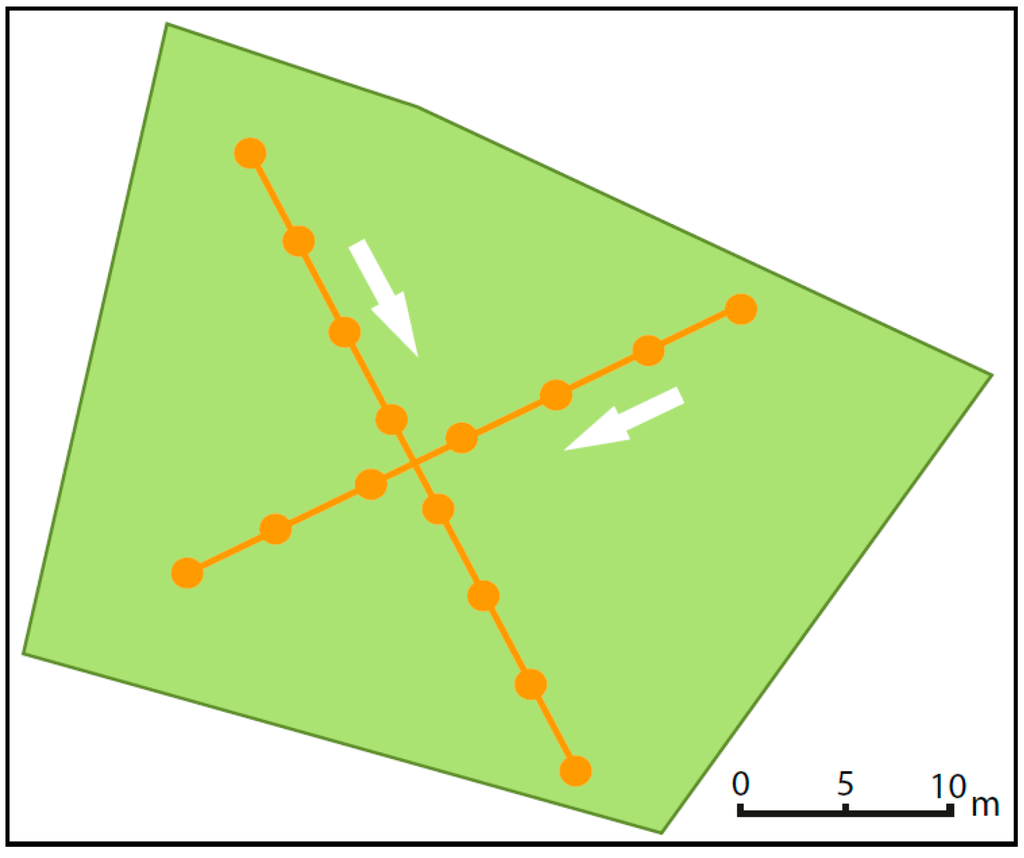
Remote Sensing, Free Full-Text

Remote Sensing in Ecology and Conservation - Wiley Online Library
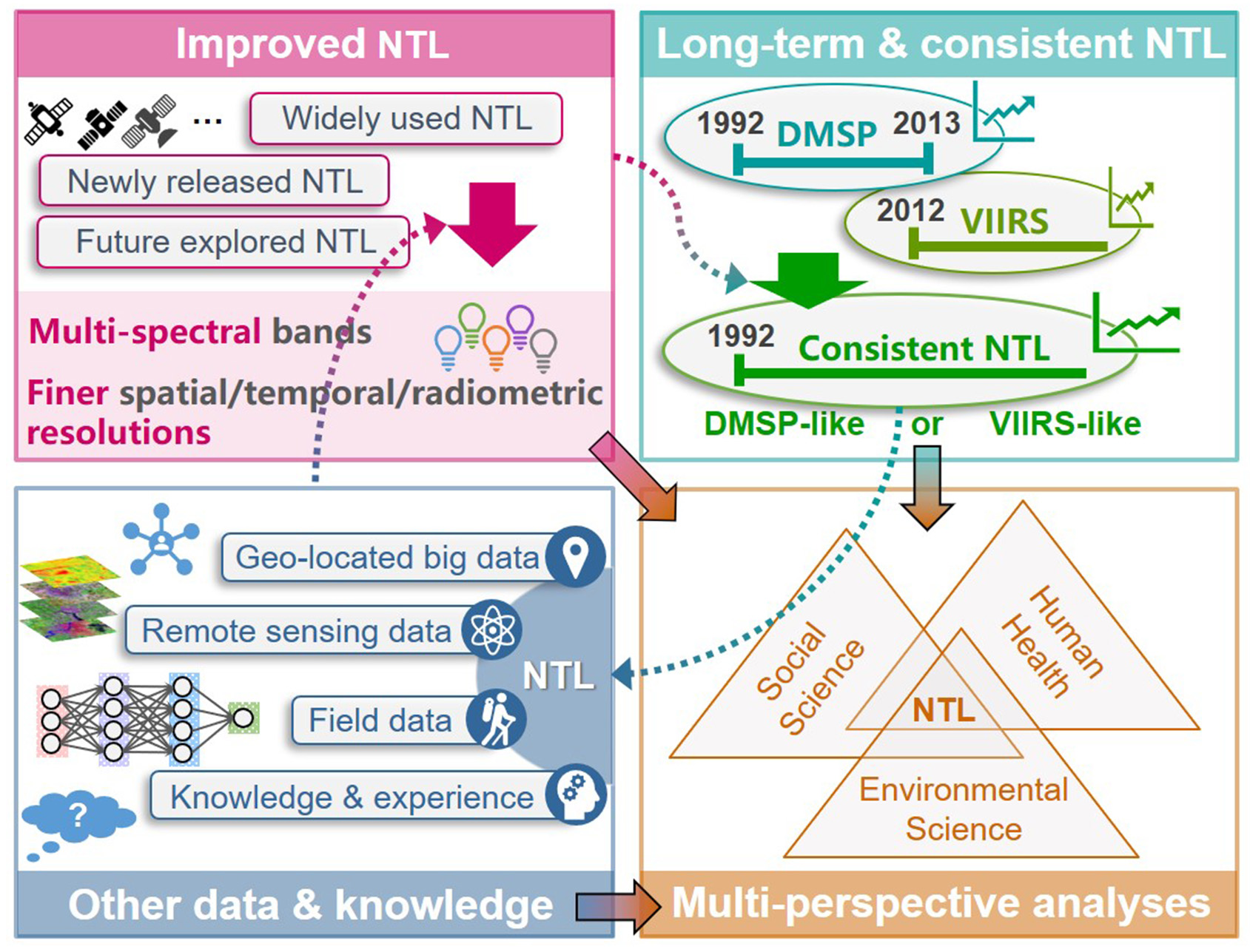
Remote Sensing, Free Full-Text
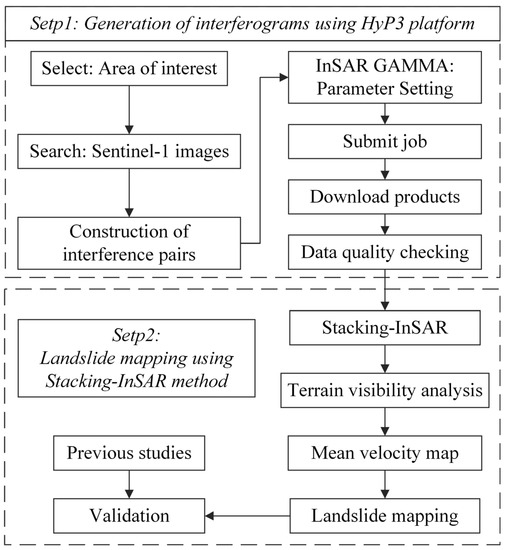
Gamma Remote Sensing Download - Colaboratory
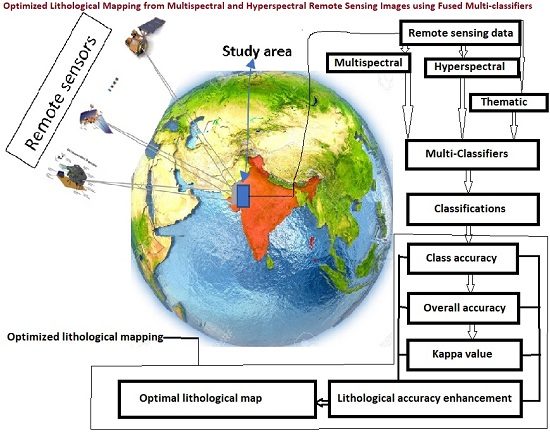
Hyperspectral Remote Sensing Data - Colaboratory
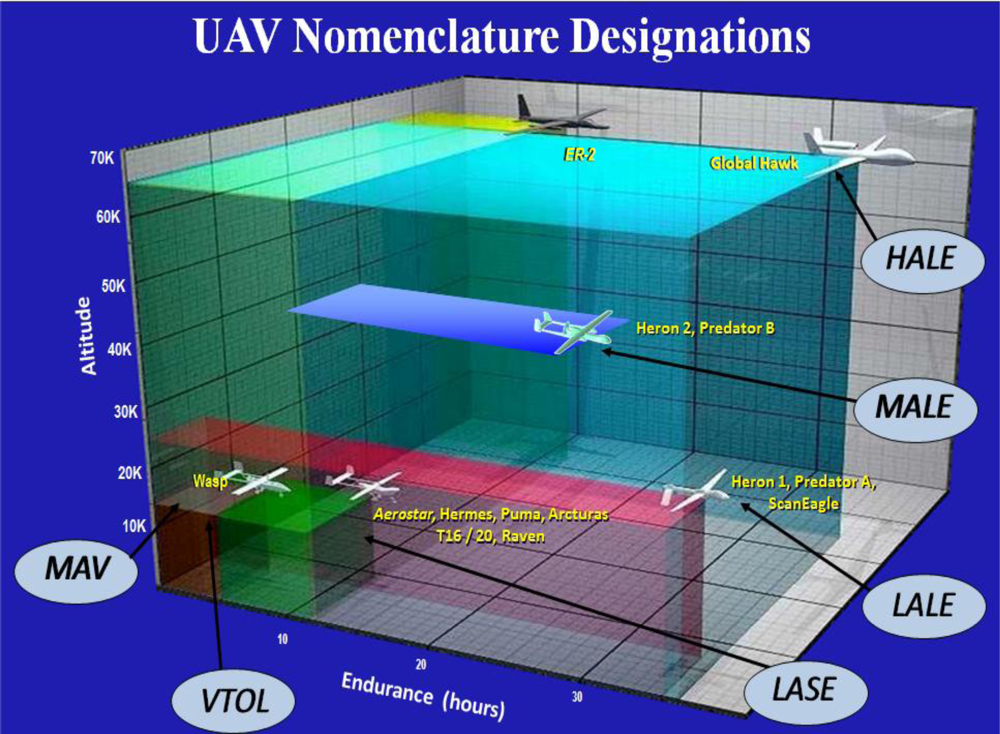
Remote Sensing, Free Full-Text
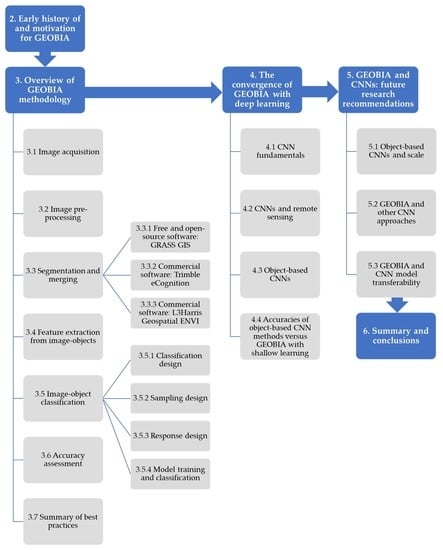
Envi Remote Sensing Software Free Full Version - Colaboratory
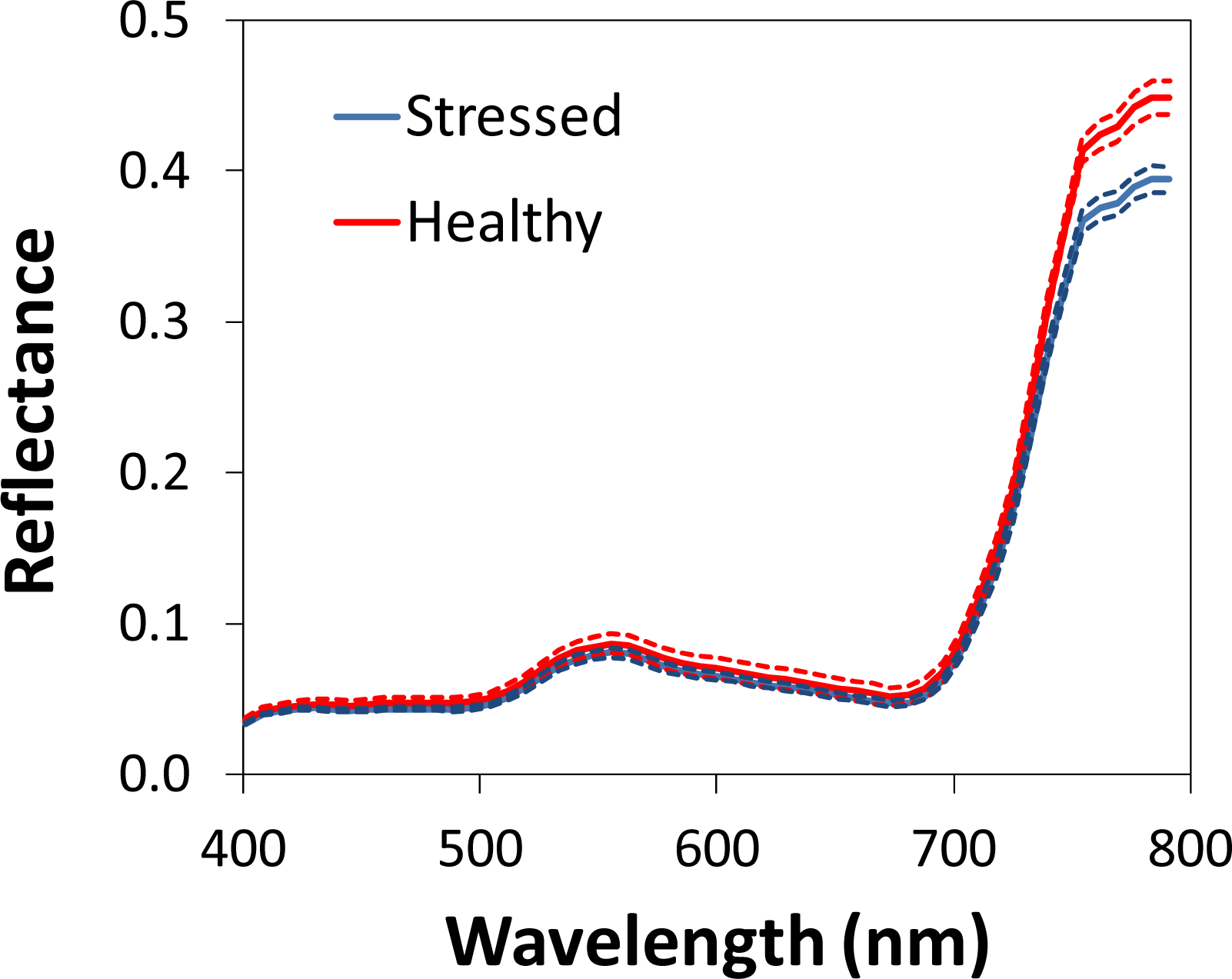
Remote Sensing, Free Full-Text
Recomendado para você
-
Legend Piece [Infinite Level] Scripts25 abril 2025
-
State of Gameplay, Sep 2021 - League of Legends25 abril 2025
-
ALL NEW SECRET *🍩UPDATE 0.5* OP CODES For LEGEND PIECE In Roblox Legend Piece codes 202225 abril 2025
-
A multifaceted gradient in human cerebellum of structural and functional development25 abril 2025
-
National Mall - Wikipedia25 abril 2025
-
Growth of photovoltaics - Wikipedia25 abril 2025
-
Create interactive charts to tell your story more effectively25 abril 2025
-
Changing the legend names on plotly express line chart - 📊 Plotly Python - Plotly Community Forum25 abril 2025
-
The SONU Sleep System Every Side Sleeper's Dream25 abril 2025
-
Special Effects IPA25 abril 2025
você pode gostar
-
Anime Kiss Anime Couple GIF - Anime Kiss Anime Couple Anime - Discover & Share GIFs25 abril 2025
-
Great Temple Rebel & Empire Tile Puzzle Solution Guide in Lego Star Wars The Skywalker Saga25 abril 2025
-
Arceus X Beta V3 Release (Next Week)#arceusx #spdmteam #SPDM25 abril 2025
-
Indiana25 abril 2025
-
2022 McDONALD'S Super Mario Bros Movie Nintendo HAPPY MEAL TOYS Or Set25 abril 2025
-
model clicks, essie gel couture longwear nail polish25 abril 2025
-
How Did They Get Young Luke Skywalker in Mandalorian? Scott Lang, Explained25 abril 2025
-
Tommy Hilfiger G-III Apparel Group, Ltd.25 abril 2025
-
PES 2022 Graphics. 🥴 📸: Trolling - Troll Football - OTF25 abril 2025
-
THE 4 BEAUTIFUL LADIES 💗 Illustration by Kei Sasuga : r25 abril 2025