AlphaDDA: strategies for adjusting the playing strength of a fully
Por um escritor misterioso
Last updated 22 fevereiro 2025
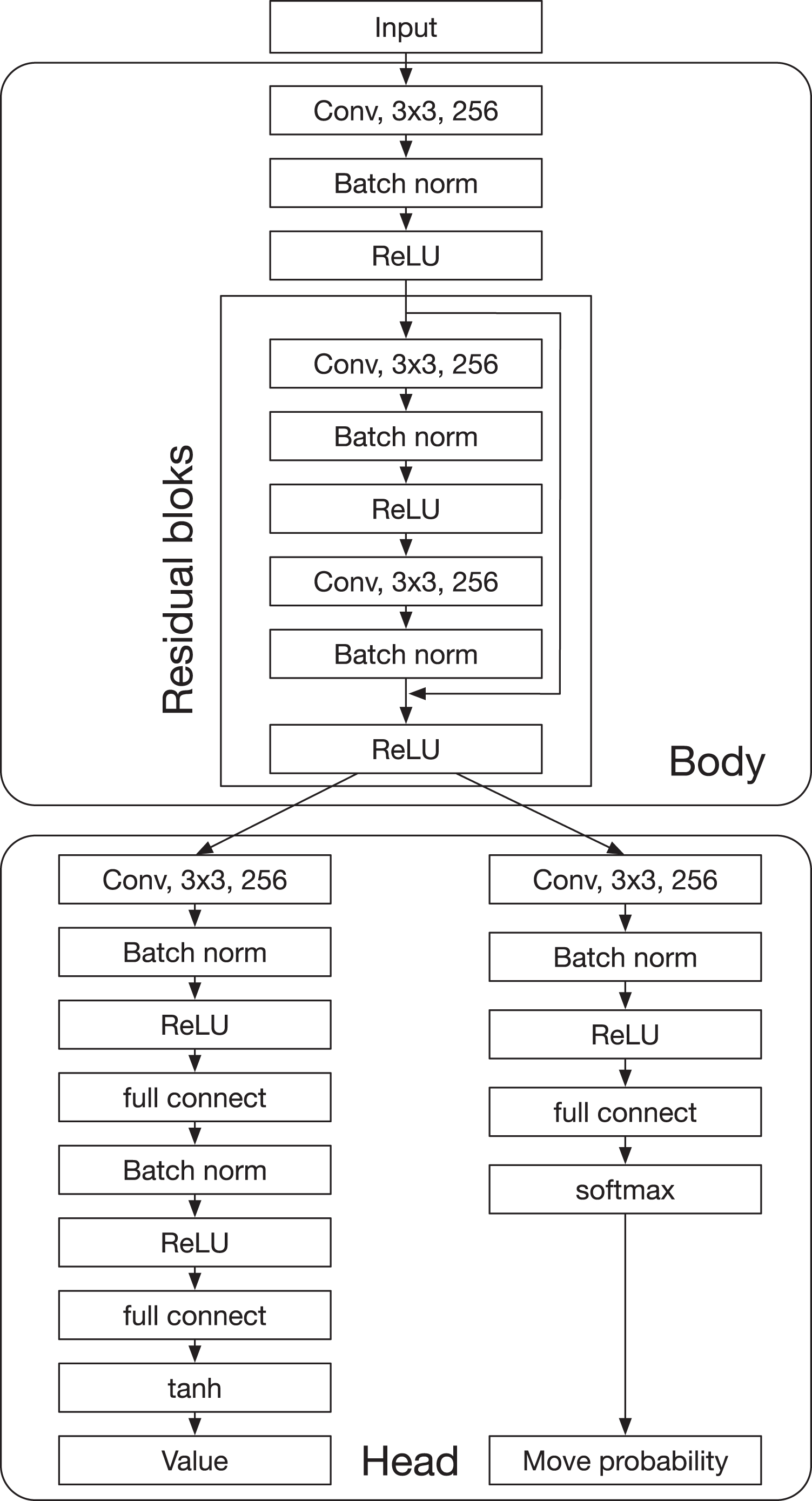
Artificial intelligence (AI) has achieved superhuman performance in board games such as Go, chess, and Othello (Reversi). In other words, the AI system surpasses the level of a strong human expert player in such games. In this context, it is difficult for a human player to enjoy playing the games with the AI. To keep human players entertained and immersed in a game, the AI is required to dynamically balance its skill with that of the human player. To address this issue, we propose AlphaDDA, an AlphaZero-based AI with dynamic difficulty adjustment (DDA). AlphaDDA consists of a deep neural network (DNN) and a Monte Carlo tree search, as in AlphaZero. AlphaDDA learns and plays a game the same way as AlphaZero, but can change its skills. AlphaDDA estimates the value of the game state from only the board state using the DNN. AlphaDDA changes a parameter dominantly controlling its skills according to the estimated value. Consequently, AlphaDDA adjusts its skills according to a game state. AlphaDDA can adjust its skill using only the state of a game without any prior knowledge regarding an opponent. In this study, AlphaDDA plays Connect4, Othello, and 6x6 Othello with other AI agents. Other AI agents are AlphaZero, Monte Carlo tree search, the minimax algorithm, and a random player. This study shows that AlphaDDA can balance its skill with that of the other AI agents, except for a random player. AlphaDDA can weaken itself according to the estimated value. However, AlphaDDA beats the random player because AlphaDDA is stronger than a random player even if AlphaDDA weakens itself to the limit. The DDA ability of AlphaDDA is based on an accurate estimation of the value from the state of a game. We believe that the AlphaDDA approach for DDA can be used for any game AI system if the DNN can accurately estimate the value of the game state and we know a parameter controlling the skills of the AI system.

Build Alpha Reviews, Trading Reviews and Vendors
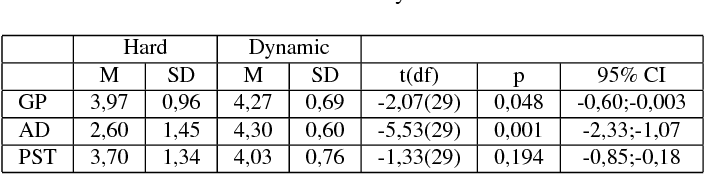
PDF] Dynamic difficulty adjustment through parameter manipulation for Space Shooter game

Slices of the (a) first (horizontal), (b) second (latteral) and (c)
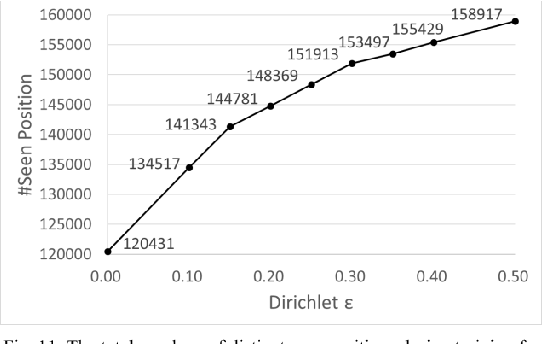
AlphaZero for a Non-Deterministic Game

The Psychology of Trading: Series 34 Exam Insights - FasterCapital

Schematic diagram of the Dynamic Difficulty Adjustment system.
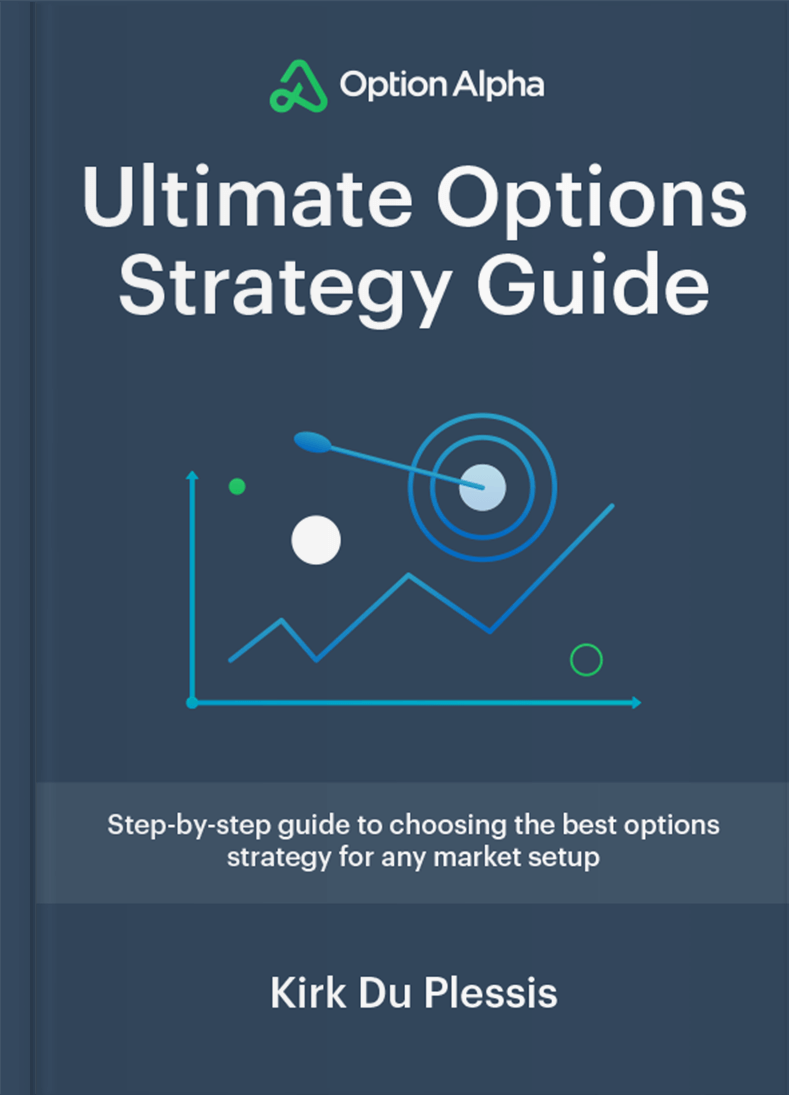
Ultimate Options Strategy Guide

Difficult flow of the player, adapted from Hunicke and Chapman [7]

Elbow plot with the mean squared error as a function of the number of

Immediate strength gains
Recomendado para você
-
Mastering the game of Go without human knowledge22 fevereiro 2025
-
AlphaZero_Connect4/README.md at master · plkmo/AlphaZero_Connect422 fevereiro 2025
-
Google跑不到谱· Issue #30 · NeymarL/ChineseChess-AlphaZero · GitHub22 fevereiro 2025
-
Time manager Alphazero - Leela Chess Zero22 fevereiro 2025
-
redis - golang Package Health Analysis22 fevereiro 2025
-
alpha-zero · GitHub Topics · GitHub22 fevereiro 2025
-
Alpha Zero will be coming back! Who will be the boss , SF 1022 fevereiro 2025
-
Alphazero baseline for the Kaggle ConnectX competition (#28422 fevereiro 2025
-
Leela Zero( A Neural Network engine similar to Alpha Zero) - Chess22 fevereiro 2025
-
AlphaZero for Backgammon · Issue #774 · google-deepmind/open_spiel22 fevereiro 2025
você pode gostar
-
Legend's Murder Mystery 2 Codes for December 2023: Credits22 fevereiro 2025
-
PANTERS™ 2222 (@PantersNFT) / X22 fevereiro 2025
-
Top Boy cast, Full list of characters in Netflix series22 fevereiro 2025
-
Code Geass: Lelouch of the Rebellion Lost Stories – Now Available in Japan22 fevereiro 2025
-
News Briefs Hospitality Technology22 fevereiro 2025
-
All in One Game Offline 2023 App Trends 2023 All in One Game22 fevereiro 2025
-
💵💎 ROBLOX LIMITEDS💎💵 📈HIGH DEMAND 📈 🔒CHEAP AND SAFE🔒 (250+ ITEMS) $975.34 - PicClick22 fevereiro 2025
-
RAGE QUIT MIGUEL HAS A BAD DAY! (Tekken 7 Season 3)- Leroy Smith22 fevereiro 2025
-
funko Pop A Nightmare On Elm Street Freddy Krueger 8-Bit Gamestop Excl22 fevereiro 2025
-
2026 World Cup logo & branding revealed by FIFA ahead of tournament in United States, Mexico & Canada22 fevereiro 2025