Computational intelligence modeling of hyoscine drug solubility and solvent density in supercritical processing: gradient boosting, extra trees, and random forest models
Por um escritor misterioso
Last updated 31 março 2025
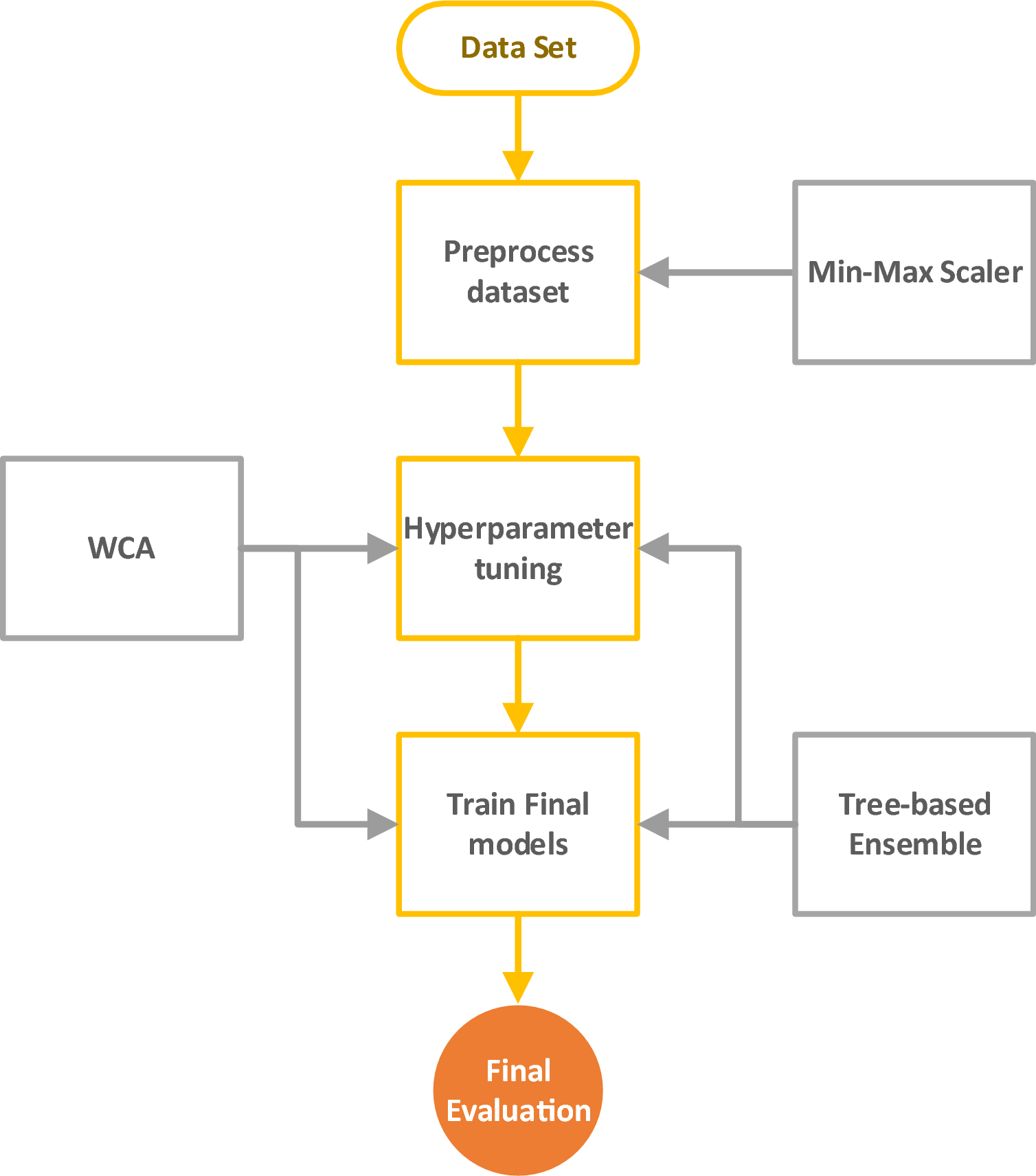
Clustering of microglia single cell transcriptomes using tSNE, PCA

Optimizing Pharmacokinetic Property Prediction Based on Integrated

Representative machine learning algorithms. Machine learning is a
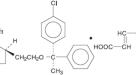
Computational intelligence modeling of hyoscine drug solubility
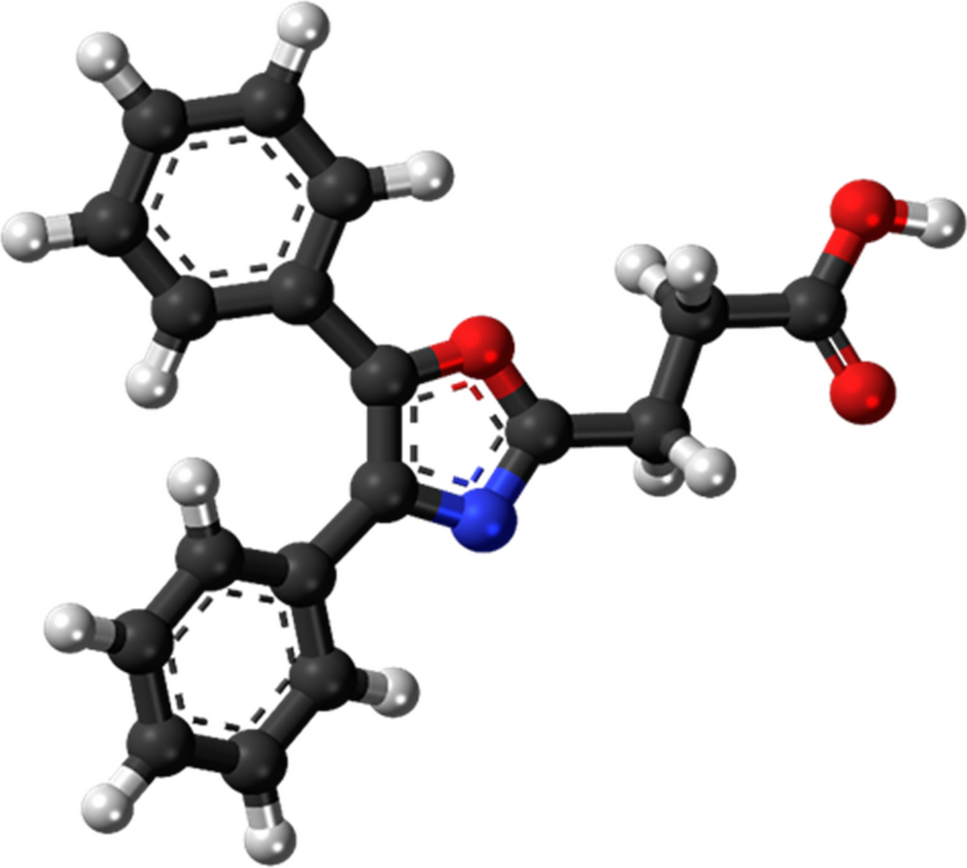
Design of predictive model to optimize the solubility of Oxaprozin
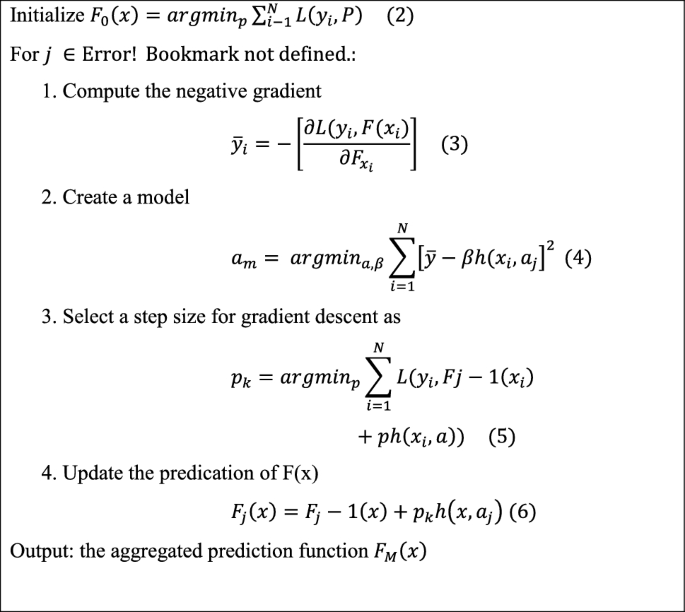
Design of predictive model to optimize the solubility of Oxaprozin
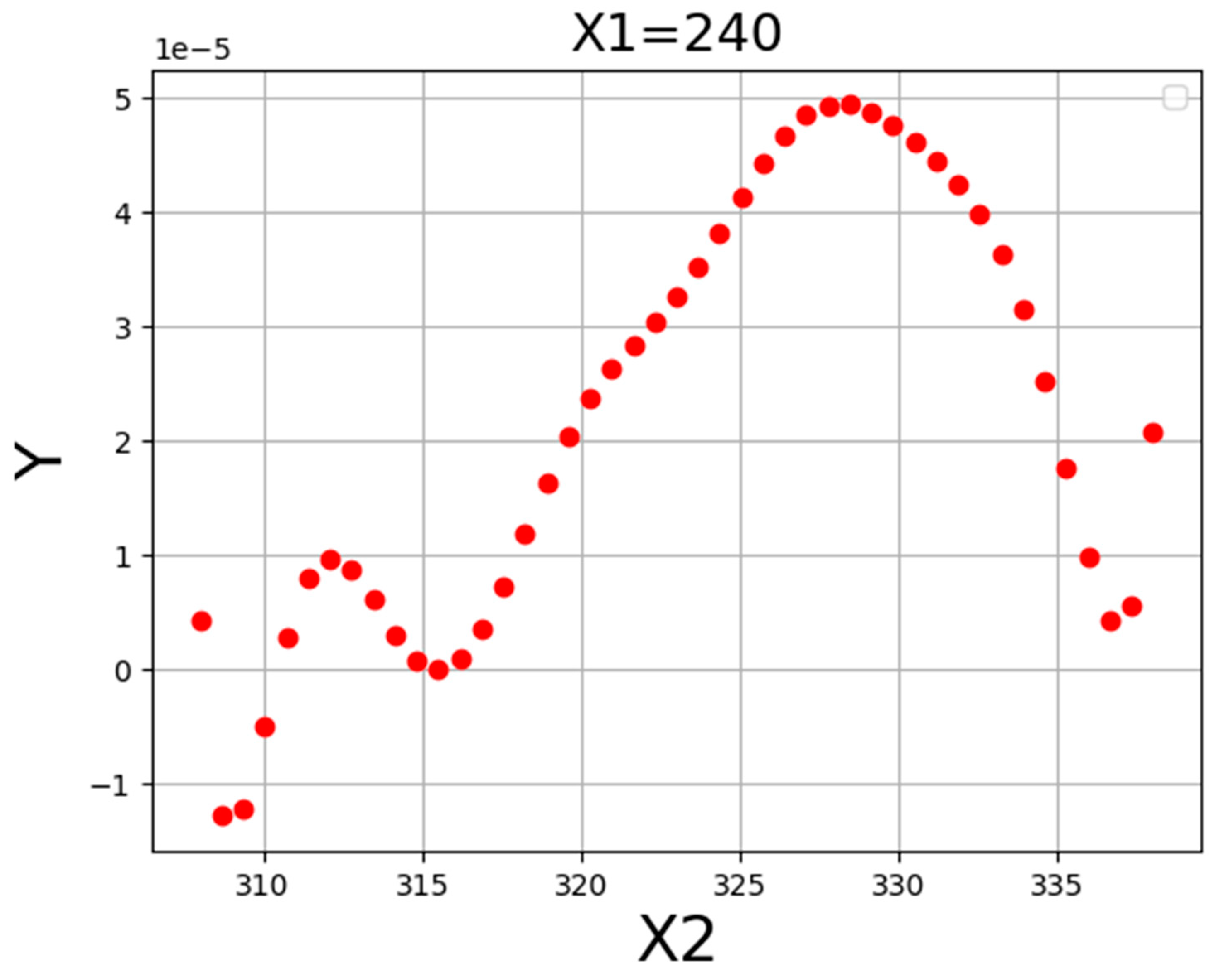
Molecules, Free Full-Text

Data distribution, P (pressure), T (temperature), and Y
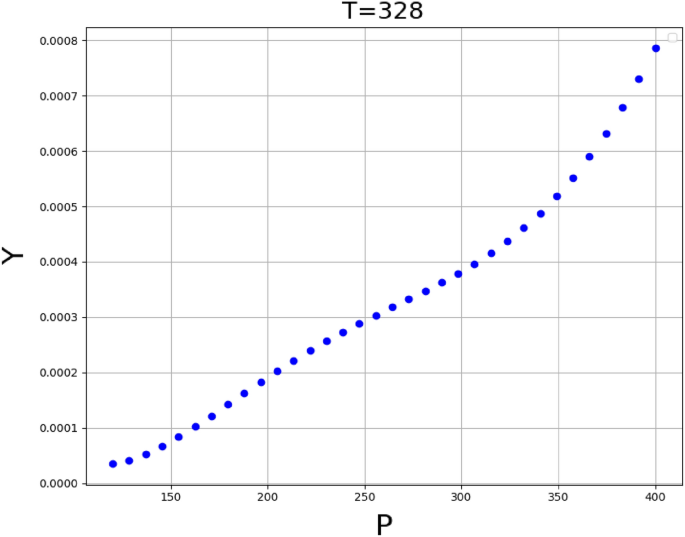
Computational simulation and target prediction studies of

Evaluation methodology based on k-fold crossvalidation.
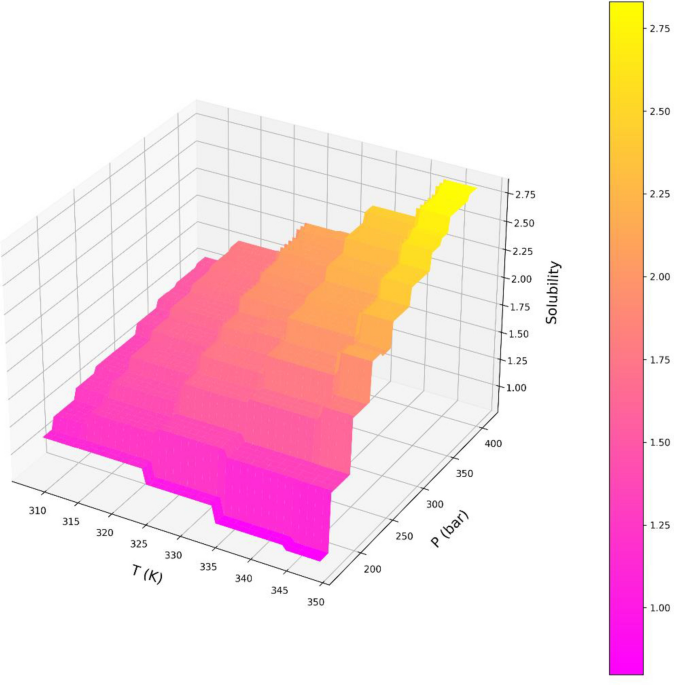
Computational intelligence modeling of hyoscine drug solubility
Recomendado para você
-
Bay Area Speedcubin' 59 LIVE - SSF 202431 março 2025
-
ACCESS for ELLs Score Report Interpretation Training on Vimeo31 março 2025
-
Ipl Secore - India 202331 março 2025
-
Applied and experimental bacterial vaccines. Today, established31 março 2025
-
The popularity has just completely exploded': Rubik's Cube's second coming, Australia sport31 março 2025
-
Enya breen hi-res stock photography and images - Alamy31 março 2025
-
CGIAR launches initiative to transform West and Central Africa's agrifood systems31 março 2025
-
A new day dawns at Westminster Christian: WCA to join the GIAA while adding football and golf, Sports31 março 2025
-
🛑South Africa vs India Hindi 2nd T20I 🛑 Live 2023 IND Vs SA CRICKET 22 GAMEPLAY31 março 2025
-
Add SOR/Kinch ranks · Issue #21 · thewca/wca-live · GitHub31 março 2025
você pode gostar
-
Miru Tights Anime's Lead Voice Actresses Sing Ending Theme in 331 março 2025
-
Subway Surfers v2.1.4 Mod (Unlimited Money) Apk - Android Mods Apk31 março 2025
-
All Forza Horizon 2 Barn Find locations31 março 2025
-
Vôlei Feminino de Louveira entra em quadra nesta quinta-feira pela31 março 2025
-
images./poster/278210779/s592/tempora31 março 2025
-
Roweb Development31 março 2025
-
The Third Saiyan - (What-If) Super Saiyan 3 but Blue? - Wattpad31 março 2025
-
Robux gratis, •, Roblox31 março 2025
-
Summer Resort - Episode 2: Tennis Menace31 março 2025
-
GitHub - Glitchii/rickroll-lyrics-PS1: Changes name and host in the terminal to a rickroll after a command.31 março 2025