Identifying common transcriptome signatures of cancer by interpreting deep learning models, Genome Biology
Por um escritor misterioso
Last updated 16 abril 2025
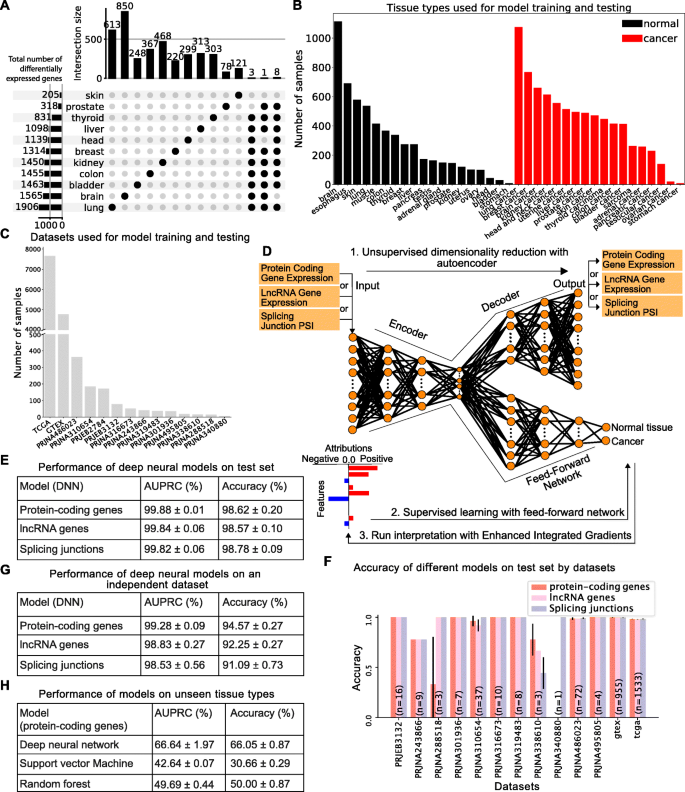
Background Cancer is a set of diseases characterized by unchecked cell proliferation and invasion of surrounding tissues. The many genes that have been genetically associated with cancer or shown to directly contribute to oncogenesis vary widely between tumor types, but common gene signatures that relate to core cancer pathways have also been identified. It is not clear, however, whether there exist additional sets of genes or transcriptomic features that are less well known in cancer biology but that are also commonly deregulated across several cancer types. Results Here, we agnostically identify transcriptomic features that are commonly shared between cancer types using 13,461 RNA-seq samples from 19 normal tissue types and 18 solid tumor types to train three feed-forward neural networks, based either on protein-coding gene expression, lncRNA expression, or splice junction use, to distinguish between normal and tumor samples. All three models recognize transcriptome signatures that are consistent across tumors. Analysis of attribution values extracted from our models reveals that genes that are commonly altered in cancer by expression or splicing variations are under strong evolutionary and selective constraints. Importantly, we find that genes composing our cancer transcriptome signatures are not frequently affected by mutations or genomic alterations and that their functions differ widely from the genes genetically associated with cancer. Conclusions Our results highlighted that deregulation of RNA-processing genes and aberrant splicing are pervasive features on which core cancer pathways might converge across a large array of solid tumor types.
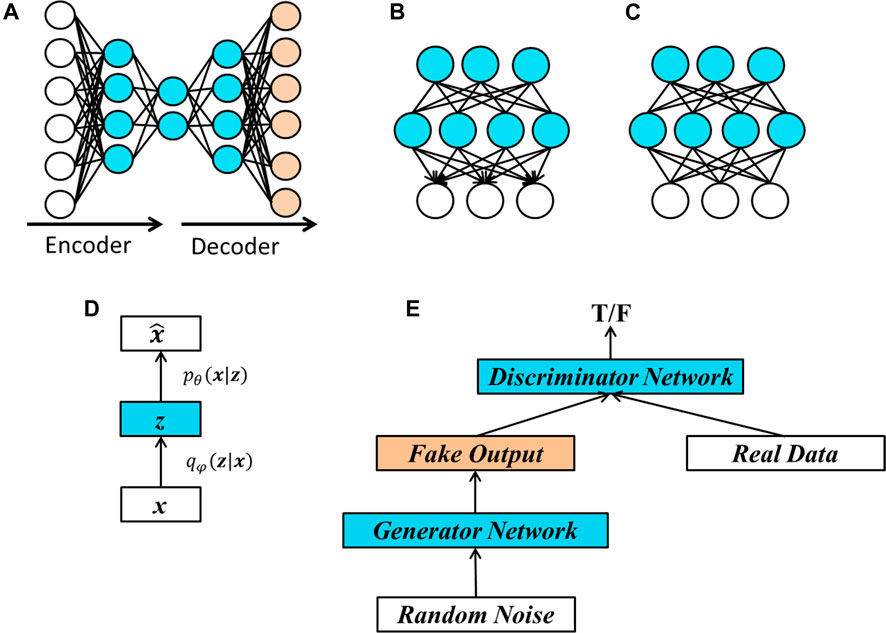
Frontiers A Brief Review on Deep Learning Applications in
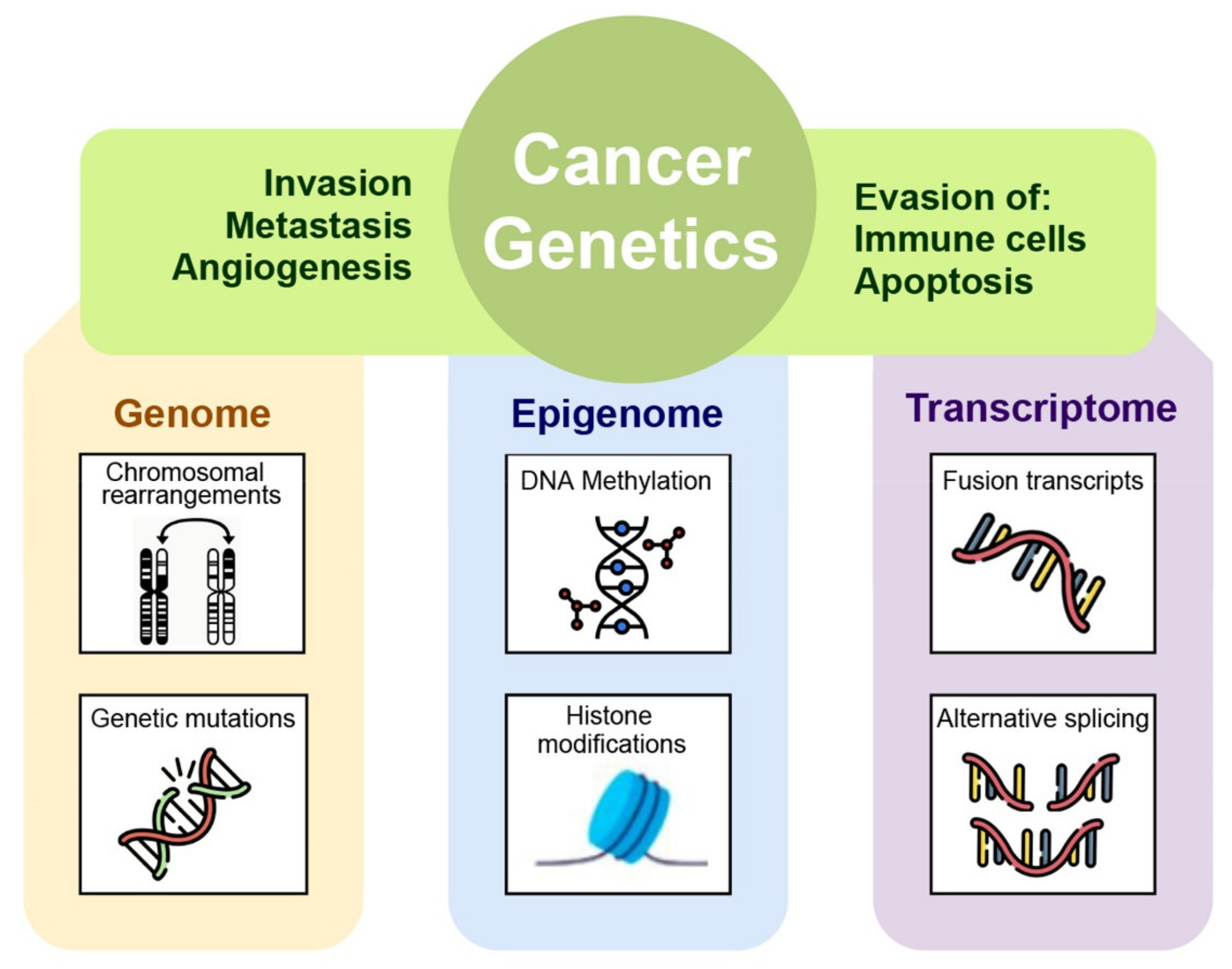
IJMS, Free Full-Text

Splicing signature database development to delineate cancer
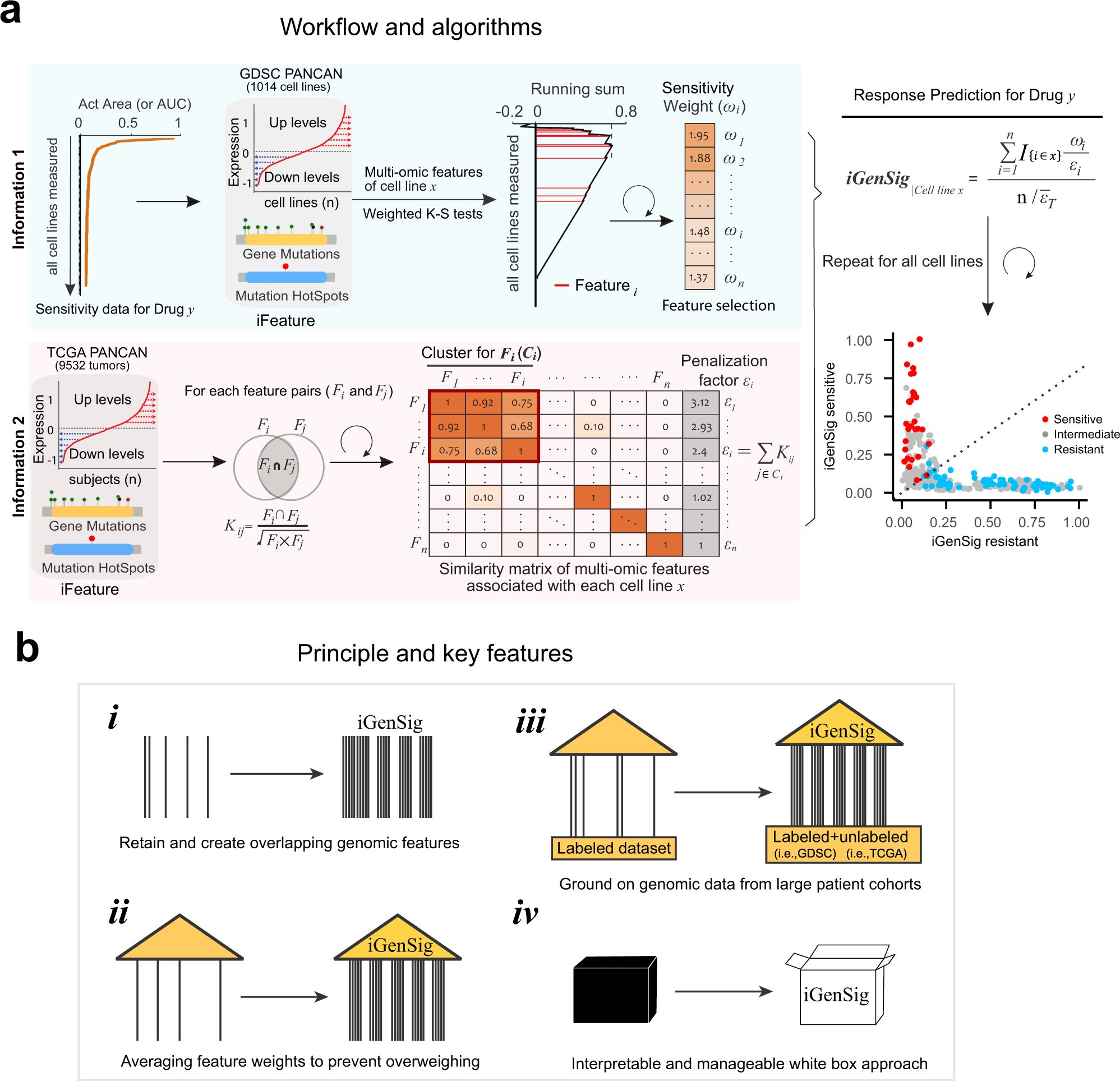
An integral genomic signature approach for tailored cancer therapy
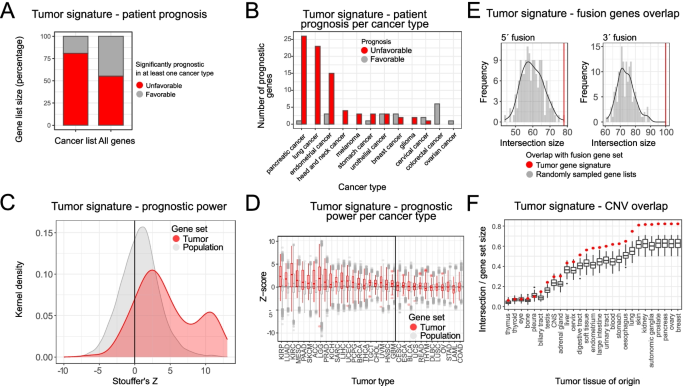
Identifying tumor cells at the single-cell level using machine
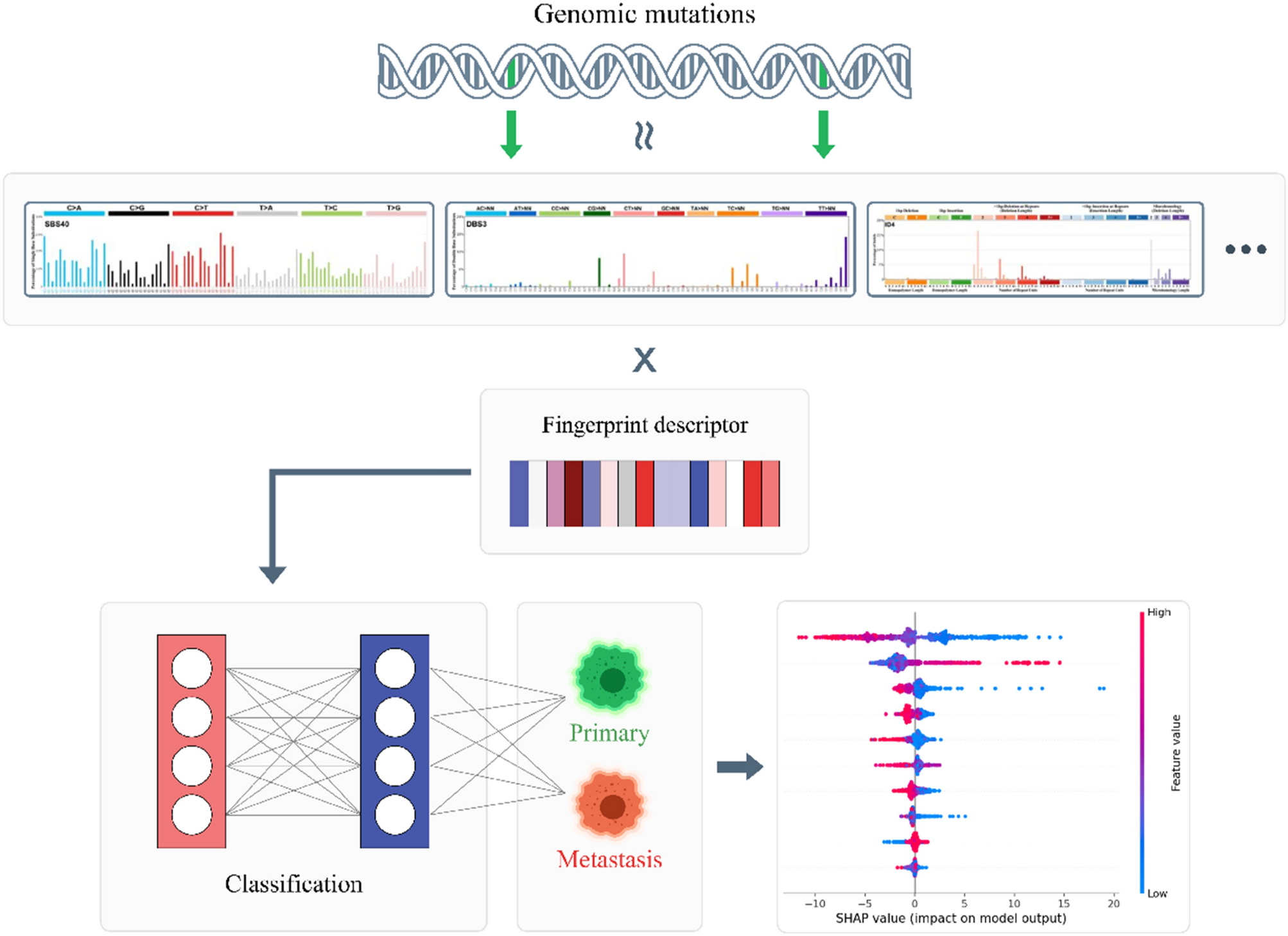
Deep learning model accurately classifies metastatic tumors from
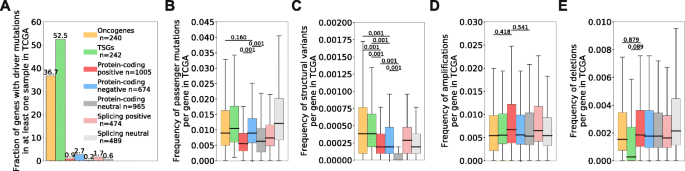
Identifying common transcriptome signatures of cancer by
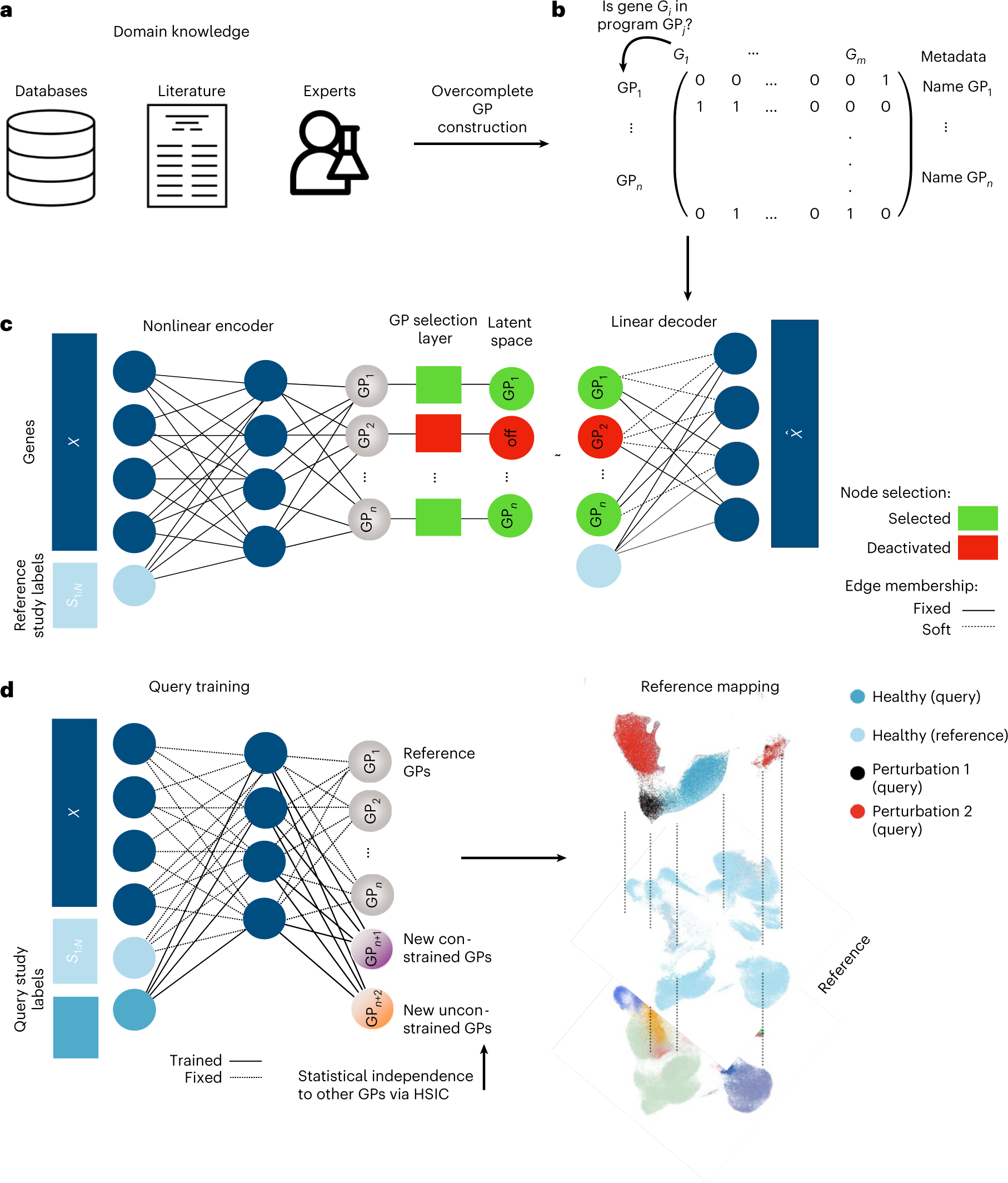
Biologically informed deep learning to query gene programs in
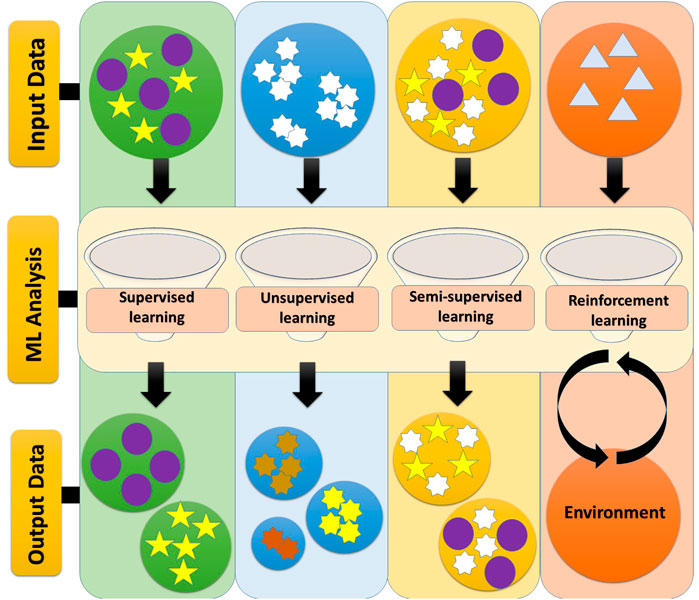
Frontiers Machine Learning: A New Prospect in Multi-Omics Data
Recomendado para você
-
SumDivide-best FREE block blasting puzzle game to test your brain::Appstore for Android16 abril 2025
-
Making a Sugar Thermometer16 abril 2025
-
Hodological organization of spoken language production and singing in the human brain16 abril 2025
-
Improving the study of brain-behavior relationships by revisiting basic assumptions: Trends in Cognitive Sciences16 abril 2025
-
Polygenic heterogeneity in antidepressant treatment and placebo response16 abril 2025
-
Ryuta Kawashima: The devil who cracked the dementia code, The Independent16 abril 2025
-
Brain and Behavior: Vol 13, No 916 abril 2025
-
Synaptic Plasticity Learning and Memory in Perirhinal Cortex16 abril 2025
-
Blinking a Book - Create Your Own Comic Strips Online with MakeBeliefsComix16 abril 2025
-
Ephaptic Interactions in the Mammalian Olfactory System16 abril 2025
você pode gostar
-
Ping Pong the Animation The Wind Makes It Too Hard to Hear (TV16 abril 2025
-
FNaF Sister Location Update #1 – OMG Girls Game!16 abril 2025
-
Aaah! Zombies!! (2007) - IMDb16 abril 2025
-
Need For Speed III Hot Pursuit [SLUS-00620] ROM - PSX Download16 abril 2025
-
Como acompanhar os jogos de futebol pelo Google em tempo real - Canaltech16 abril 2025
-
Aphelios ARAM Build - Best Guide and Runes for Aphelios on Patch 13.2416 abril 2025
-
Tv Jogos, Jogos da Barbie16 abril 2025
-
Friv Games #1 - FireBoy & WaterGirl16 abril 2025
-
Formulário para adesão de Municípios ao convênio NFS-e — Portal da Nota Fiscal de Serviço eletrônica16 abril 2025
-
Vlog: Study Abroad Tips for First Generation College Students16 abril 2025