DREAMS: deep read-level error model for sequencing data applied to
Por um escritor misterioso
Last updated 25 abril 2025
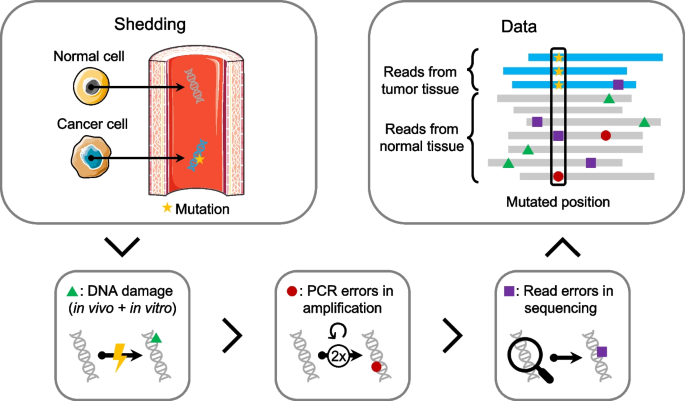
Circulating tumor DNA detection using next-generation sequencing (NGS) data of plasma DNA is promising for cancer identification and characterization. However, the tumor signal in the blood is often low and difficult to distinguish from errors. We present DREAMS (Deep Read-level Modelling of Sequencing-errors) for estimating error rates of individual read positions. Using DREAMS, we develop statistical methods for variant calling (DREAMS-vc) and cancer detection (DREAMS-cc). For evaluation, we generate deep targeted NGS data of matching tumor and plasma DNA from 85 colorectal cancer patients. The DREAMS approach performs better than state-of-the-art methods for variant calling and cancer detection.
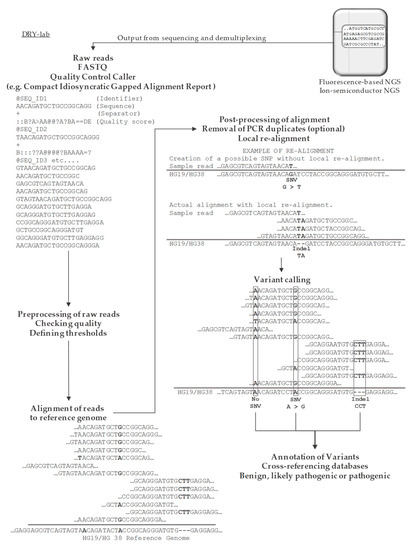
Cancers, Free Full-Text

Jesper Berg Nors - Research - Aarhus University
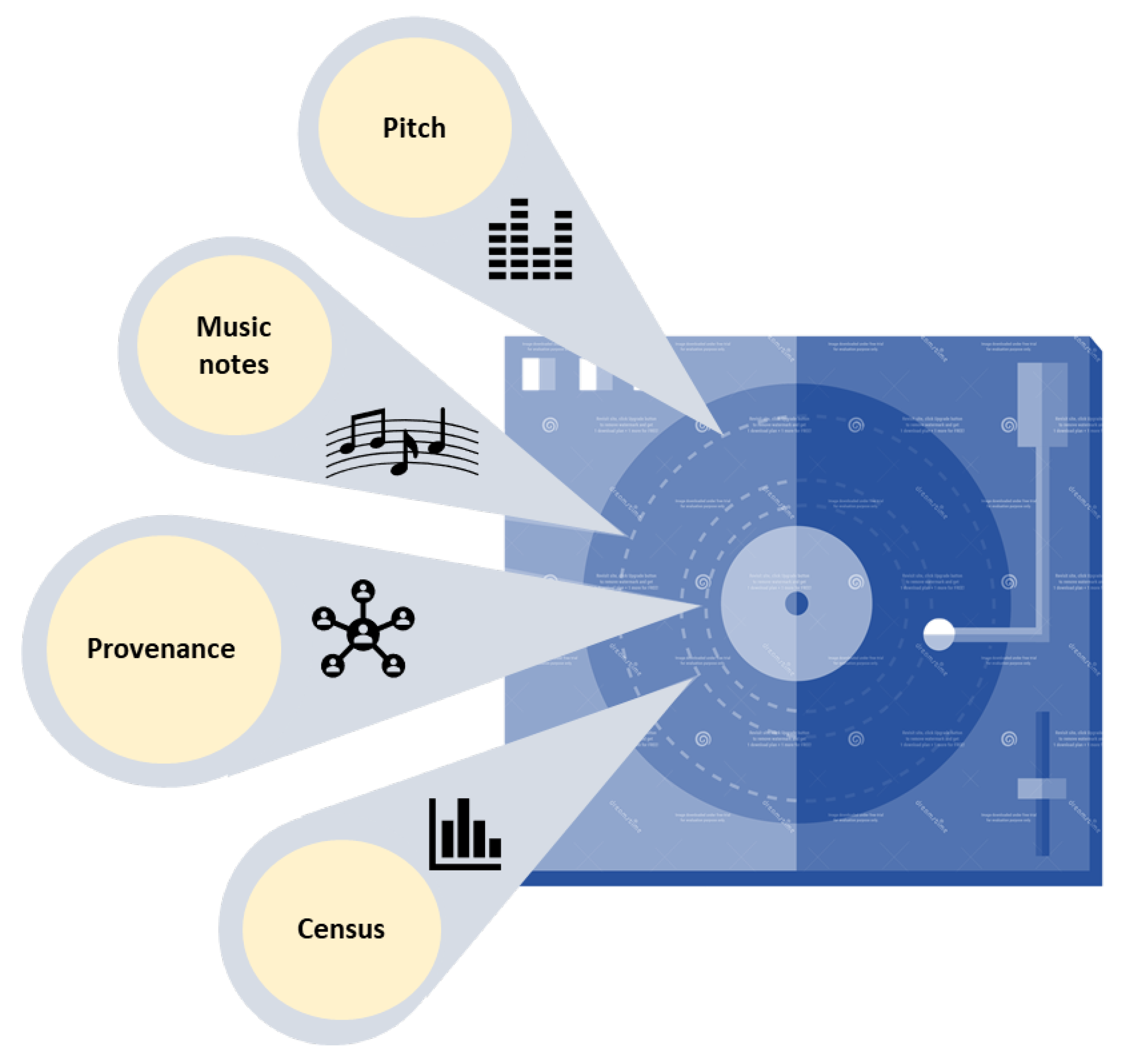
Applied Sciences, Free Full-Text
Reimagining our futures together: a new social contract for education

PDF) DREAMS: deep read-level error model for sequencing data
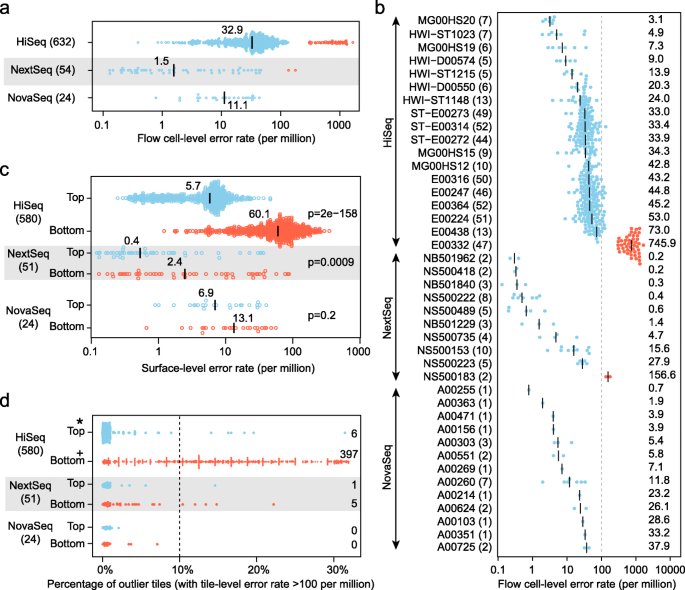
SequencErr: measuring and suppressing sequencer errors in next

Stop Stealing Dreams. if you don't underestimate me, I…
Active Site Sequence Representations of Human Kinases Outperform
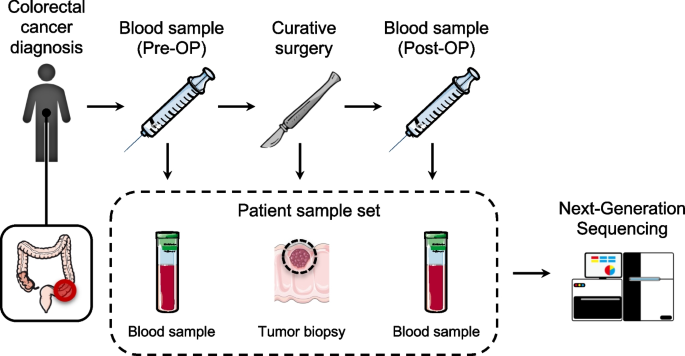
DREAMS: deep read-level error model for sequencing data applied to
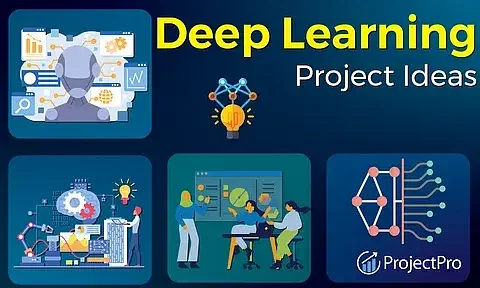
20+ Deep Learning Projects for Beginners with Source Code
Recomendado para você
-
SCP-7143-J, the crude cookie, AKA Cookie Cosplay : r/SCP25 abril 2025
-
7143-J SCP Foundation [RUS] Amino25 abril 2025
-
Banging on the Door, SCP Foundation25 abril 2025
-
press F for 7143-J : r/DankMemesFromSite1925 abril 2025
-
REDACTED] — I would fuck the shit out of that doorknob.25 abril 2025
-
open_science_fellowship_project/resources/example_data/derivatives/freesurfer/sub-01/scripts/recon-all.local-copy at master · PeerHerholz/open_science_fellowship_project · GitHub25 abril 2025
-
Miscellaneous SCPs, SCP Meta Wiki25 abril 2025
-
Neuroanatomical organization and functional roles of PVN MC4R pathways in physiological and behavioral regulations25 abril 2025
-
PDF) Tractographical model of the cortico-basal ganglia and corticothalamic connections: Improving Our Understanding of Deep Brain Stimulation25 abril 2025
-
Gorefield - Parte 2 [SCP-3166] #gorefield #garfield #scp3166 #scp #cr25 abril 2025
você pode gostar
-
A hoard of picrews/avatar creators for your MC needs : r/TheArcana25 abril 2025
-
Jogos de Tabuleiro Online - Dominó, Xadrez, Damas - دانلود APK برای آندروید25 abril 2025
-
London Chess Classic: Winners and Losers25 abril 2025
-
New fruit and veg freezing plant inaugurated in Almeria25 abril 2025
-
Jogo De Xadrez Dobrável Magnético 3 em 1 Dama Tabuleiro Portátil25 abril 2025
-
Create a Parappa Character Tier List - TierMaker25 abril 2025
-
File:Boruto logo.png - Wikimedia Commons25 abril 2025
-
Does Sony's Playstation VR 2 work on a PC?25 abril 2025
-
Friday Night Funkin Dowload iOS/Android - How to Download FNF25 abril 2025
-
Brasil Em 5 De Julho De 2021. Nesta Ilustração Fotográfica Paris25 abril 2025